The rise of “data-first” companies
Data-intensive technologies are on the rise, but many industries lack the ability to leverage data tools to stay relevant.
by Sridhar Iyengar
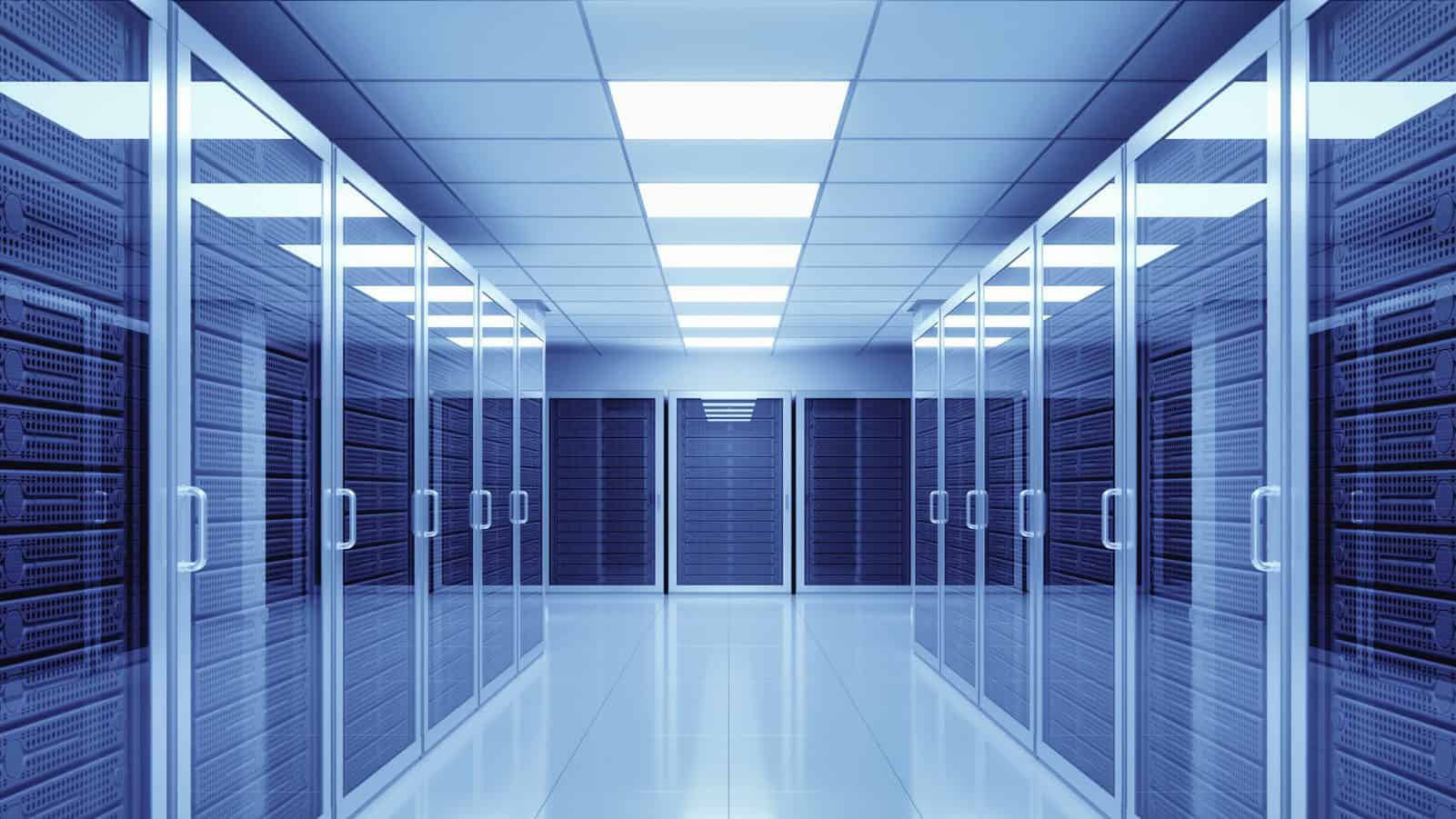
If you read most tech blogs or publications, chances are you’ll see the terms Machine Learning (ML), IoT (Internet of Things), or Synthetic Biology (SynBio) at least half a dozen times. Though these fields have been around for decades in some shape or form, it is only recently that building applications, products, and services have become affordable enough to begin wide-spread adoption. In this article, I’ll explore a few examples of how we see these new technologies coming into the market and how companies can prepare for and leverage new opportunities that concomitantly arise.
The most tangible examples of new technology invading our daily lives has to be that of connected devices – or the Internet of Things as it’s often called. The massive amounts of data sourced through IoT is presenting incredible opportunities for all industries, and ideological problems for many businesses in general. The ability to communicate with remote machines, devices, and systems has opened up new efficiencies as well as created new business models. One of my favorite examples is how companies that may not have been traditionally seen as technology companies are in fact embracing connectivity solutions to create new value and benefit for themselves and their customers.
For example, companies such as Caterpillar and John Deere have IoT systems in place that monitor their tractors and specific systems/parts to let them know when they need to be changed or to warn of any potential malfunctions. This lets the machine vendors offer servicing and maintenance more efficiently and at a lower cost, since they can predict their upcoming work orders more readily, and also allows for their customers to have less down time and fewer issues with their equipment – prevention is often much cheaper than cure. What’s important to note here is that this is an infrastructure play – the end consumer or user is rarely aware of what’s happening behind the scenes (or literally under the hood in this case).
In addition to digital technologies, the arsenal of biological tools is also expanding at an exponential rate. Synthetic Biology has been receiving its fair share of the spotlight as well. The field of SynBio involves manipulating biological systems to perform various tasks much like the way a computer scientist would program a computer – anything from manipulating DNA using CRISPR to try and create life-saving therapies, to using yeast or bacteria to produce new proteins and materials, to growing meat and other food products in labs.
One of the more common applications in SynBio is to use organisms like yeast or bacteria to act like small factories to produce new molecules. Insulin is probably one of the most well-known drugs that’s made by such a process – the DNA that encodes for the insulin protein is inserted into bacteria that then read this DNA and form the insulin protein. Today, there are many exciting startups using this basic technique to create anything from spider silk (without spiders), milk (without cows), and eggs (without chickens).
While on the surface it may seem that IoT and SynBio are not that closely related, they share a very strong common link – namely that both fields generate and consume massive amount of data. For IoT, this is fairly easy to see – sensors and digital communications between devices. For SynBio, it may be harder to see the role of data, but in fact, I would argue that there is far more data to be dealt with since the foundation of SynBio is understanding the genome of virtually all living creatures. Furthermore, the synthesis and manufacture of biological products involves massive amount of real-time process data to keep quality control during production. The link is more obvious since both fields must rely on data science and computational technologies to help navigate through myriad data sets.
When talking about data science, “Artificial Intelligence” and “Machine Learning” are used often in popular journalism, but what exactly do these terms mean? Artificial intelligence (AI) has generally been used broadly to refer to the ability of computational systems to mimic the apparent cognitive functions of human beings – or, in other words, to be able to learn and understand information similar to how a human does. Machine Learning is a subset of AI that specifically focuses on enabling computers to learn from data without being directly programmed to do so. One of my favorite definitions of ML is that it is really just “automated statistics”. What this means is that from large data sets, you can extract trends and, in the case of a remotely-monitored tractor, see what the likelihood of something breaking down might be, or in the case of genomics, where a particular gene may be located or how it may get expressed. With such large data sets, it’s nonsensical to examine individual data points – the value is in the aggregate picture and that’s exactly what ML excels at – and that’s exactly what is needed to ingest, analyze, and make sense of the large data sets that new fields like IoT and SynBio are generating.
While it’s no surprise that we live in an increasingly data-driven world (recent reports suggest that 90% of the whole world’s data was generated in the last two years!), this nonetheless gives rise to organizational challenges about how companies can handle and leverage this abundance of data. We are in a world where the cost of collecting data is rapidly falling, and the trap that I have seen companies fall into is one where data are collected without a clear strategy for utility. In other words, the sentiment has often been “We can collect all of this data easily, so we will.” The risk is that an organization can quickly become overwhelmed by data and lose sight of its value. The classic examples that come to mind are the first generation of companies in the late 90’s and early 2000’s that combined ML and genomics to build bioinformatics products. That was a classic case of building products on top of rapidly growing data sets without really having a sense of what the true value of the data would be. Unfortunately, most of those companies are no longer around since they fell squarely into the aforementioned data trap.
What we’re seeing today is a new generation of companies that I call “data-first organizations”. What this means is that the company puts data science, analytics and IT at the center of its business and then applies these core skills to certain products and verticals. Amazon is an obvious paragon of such an organization – they analyze and adjust pricing thousands of times per second – creating a pseudo-stock market founded on their data analysis. But here’s the clever part – they are really a data-first company that just happens to be a consumer goods retailer. Understanding their data is so much at the center of their business that they built their own data infrastructure and eventually offered up that capability as a product called AWS (Amazon Web Services).
We’re seeing similar patterns across all industries – the cost of data collection is rapidly falling, and so competing companies must find ways to harness this data in new ways to remain relevant. Circling back to the previous examples, we’re seeing companies like Caterpillar and John Deere transforming into data-first companies that just happen to make heavy machinery. SynBio companies are investing heavily into data science, and they just happen to do biology – companies like Gingko Bioworks, Emerald Cloud Labs, and Transcriptic, to name a few. All of these companies have complex and sophisticated data ingestion, storage, analytics, and visualization capabilities that are the backbone of their operations.
So given all of these great advances being made in how ML and data science is used in different fields, it should be obvious to any organization that bringing a data-first strategy is paramount. Unfortunately, knowing what direction to move in and figuring out how to change organizational inertia to get there are two very different things. More traditional companies have to fight years of company culture and infrastructure impediments, often with limited success.
The biggest impediment to becoming a data-first company is how leadership responds when the collected data contradicts intuition. All too often I’ve seen leaders dismiss data in favor of their own beliefs and biases. While there are certainly times when intuition will trump everything else, anytime data and intuition are at odds is a remarkable opportunity for innovation.
Throughout history, innovation (and indeed invention itself) has happened when data and intuition are at odds. Put another way, it’s when something unexpected happens.
Let’s take a minute to reflect on this: we now live in a world where data is accessible from so many different sources – be it via connected devices or from gene sequences – and we have the tools to analyze this data to find seemingly hidden patterns. We have more opportunities than ever to challenge our intuition and beliefs – to observe the unexpected. By embracing a data-first approach, companies have a much greater opportunity to constantly challenge their inherent biases, beliefs, and intuition with constantly updated data from their operations. Indeed, this is why companies like Amazon and Caterpillar remain competitive – they share this common thread of challenging their beliefs with data. It’s the only way to stay competitive.
Data doesn’t lie.