The (unreasonable) efficiency of mathematics in business
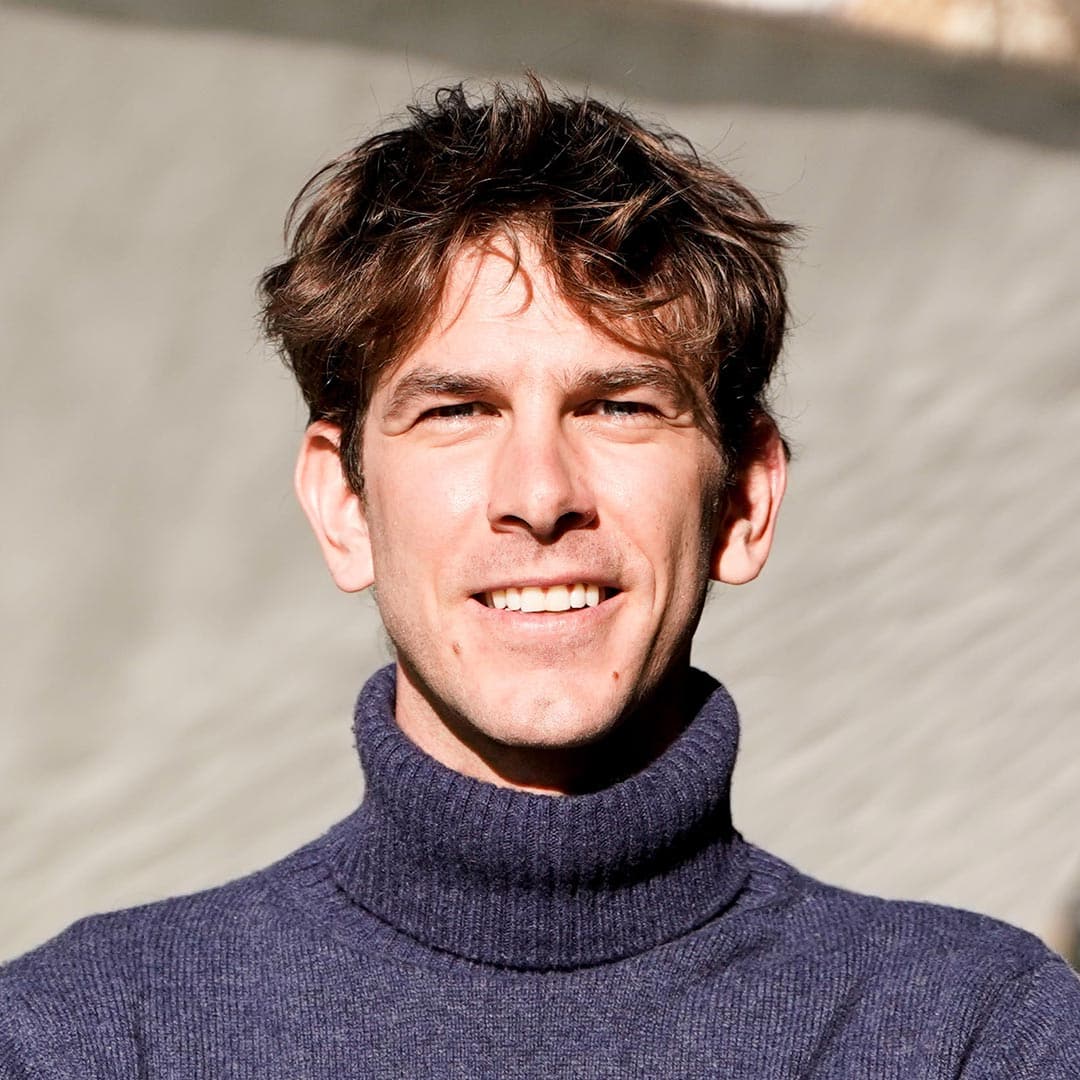
Gilberto Pastorella
Manager, business designer
Research and insights
2022
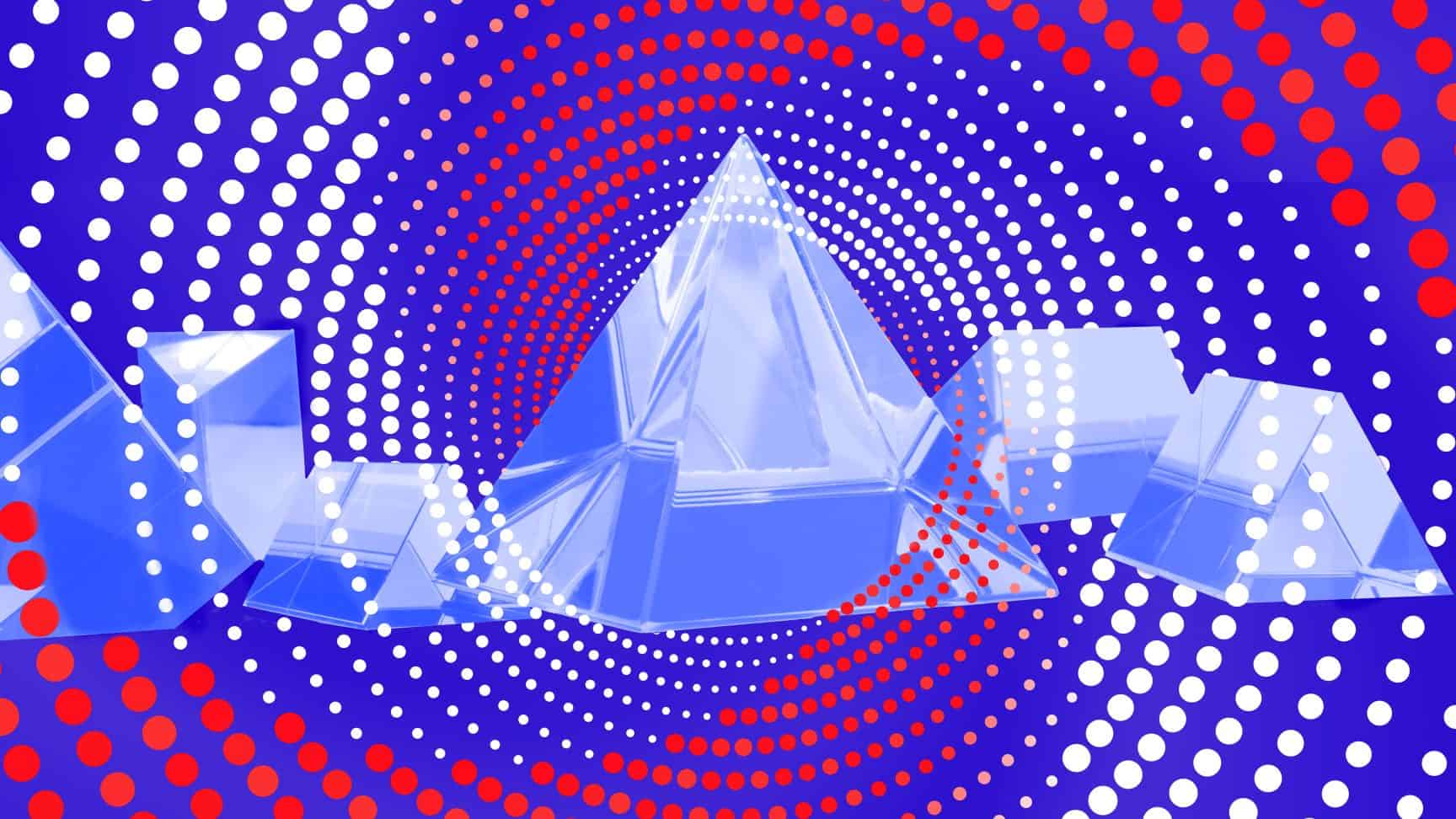
Fast forward some sixty years. What we might discuss today in a similar, hypothetical (and, most likely, virtual) conference might be the “unreasonable efficiency of mathematics in business” — “unreasonable” being once again used to say “incredible”, “bewildering”.
Still, such an adjective might put turnout at risk. Copywriters these days usually employ more direct communication methods and less ambiguous narratives.
As an example, over the past few years, Amazon’s digital shelves have accommodated books like “The ten equations that rule the world”, “Algorithms for a new world”, “The Master Algorithm”, and texts with other less-fancy, non-splashy titles.
And the algorithms they mention? Not Maxwell’s equations or Newton’s law, such as in Wigner’s case, of course: today it’s all about machine learning, AI, autonomous driving, etc.
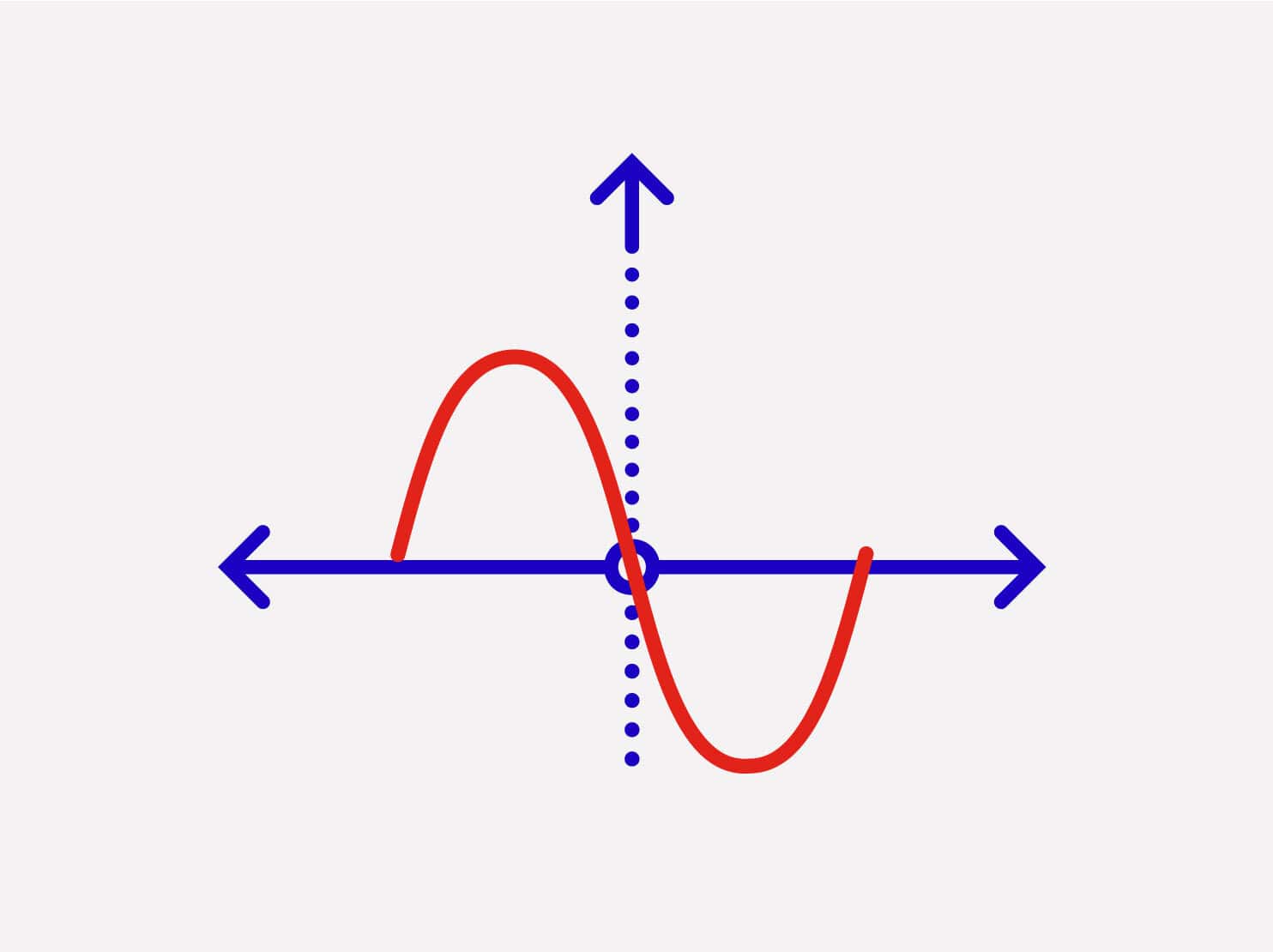
Regardless of the books’ titles or their publishing year, however, there is a fil rouge connecting most of the texts mentioned above, which also comes back in many of the discussions we have with our clients and colleagues: how can mathematics (and all its offshoots, from AI to all things IT) be so efficient across its many applications? And is that even true?
The short answer is yes. Math is not a chimera; it is not fake news. It really does work, and we have a billion examples to prove it. The more detailed answer tries to deal with how math is so efficient in business, the areas in which it comes in the handiest, and those where, on the other hand, it doesn’t always get to the hoped results.
The projects where mathematics serves business well usually focus (or should focus) on some key features:
- people and processes;
- the actual problem;
- data;
- technology;
- math itself.
If we remove or fail to consider any of these ingredients with sufficient attention, math will inevitably lead to poorer results. So let’s take a better look at each of them.
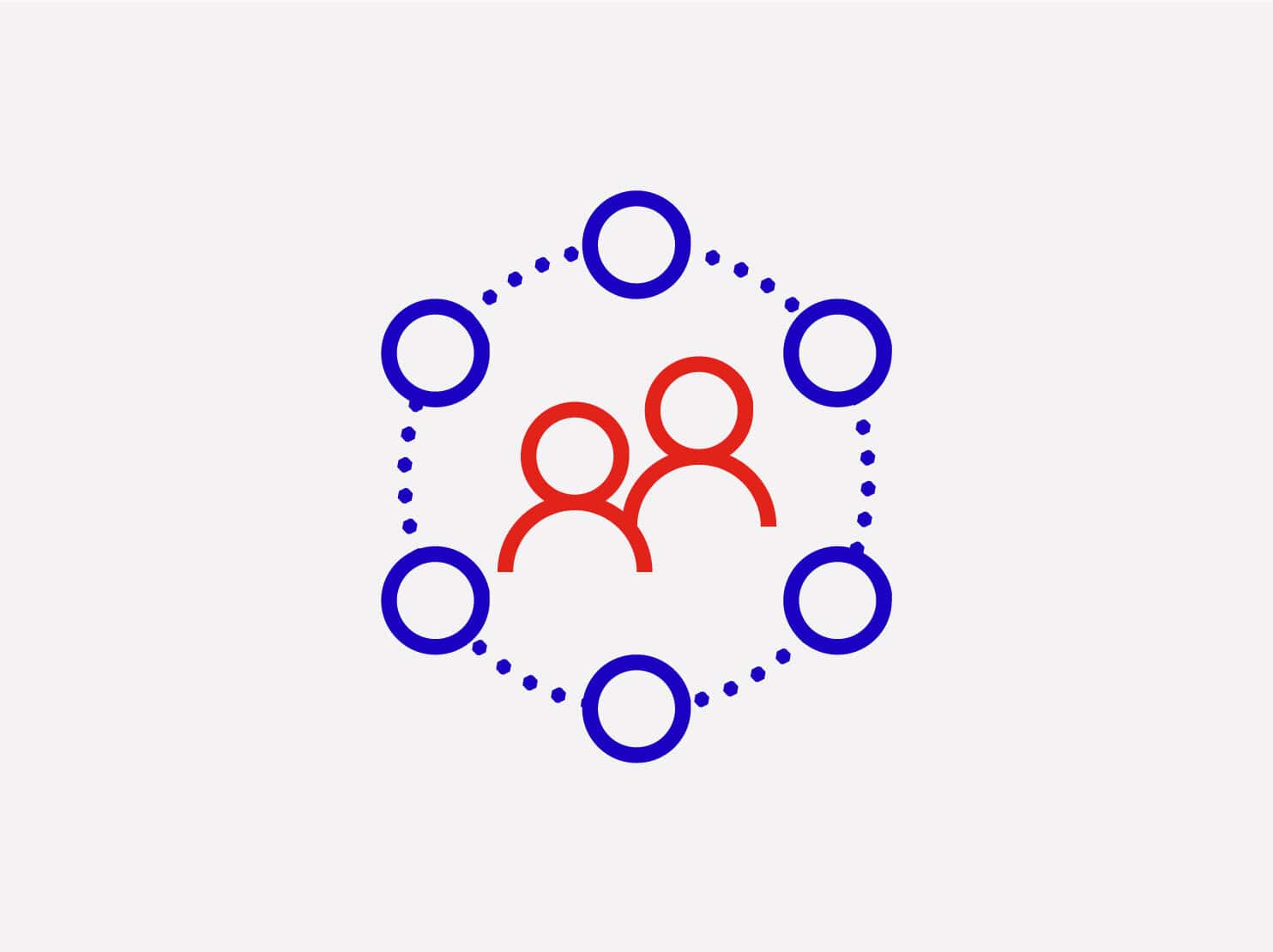
People and processes
Company culture and a correct training path for people are two essential aspects in the successful implementation of AI-related (or, more broadly, math-driven) projects to support the business. To move beyond POCs (proof of concept) and research projects, business and tech departments need to have constant communication. This is a company culture issue, whose solution also includes healthy cross-pollination and proper training — not technical training, as not everyone will become a data scientist overnight, but a mindset shift. It’s about adapting to a new way of thinking, and, effectively, a new world.
A world where an algorithm is capable of beating the chess world champion, or writing a full article starting from a quick brief. Wigner had it easy back then because he spoke to researchers in the same field. It is much harder to make this unreasonable efficiency understandable now, to a much more heterogeneous population, i.e. the one that makes up most of today’s companies.
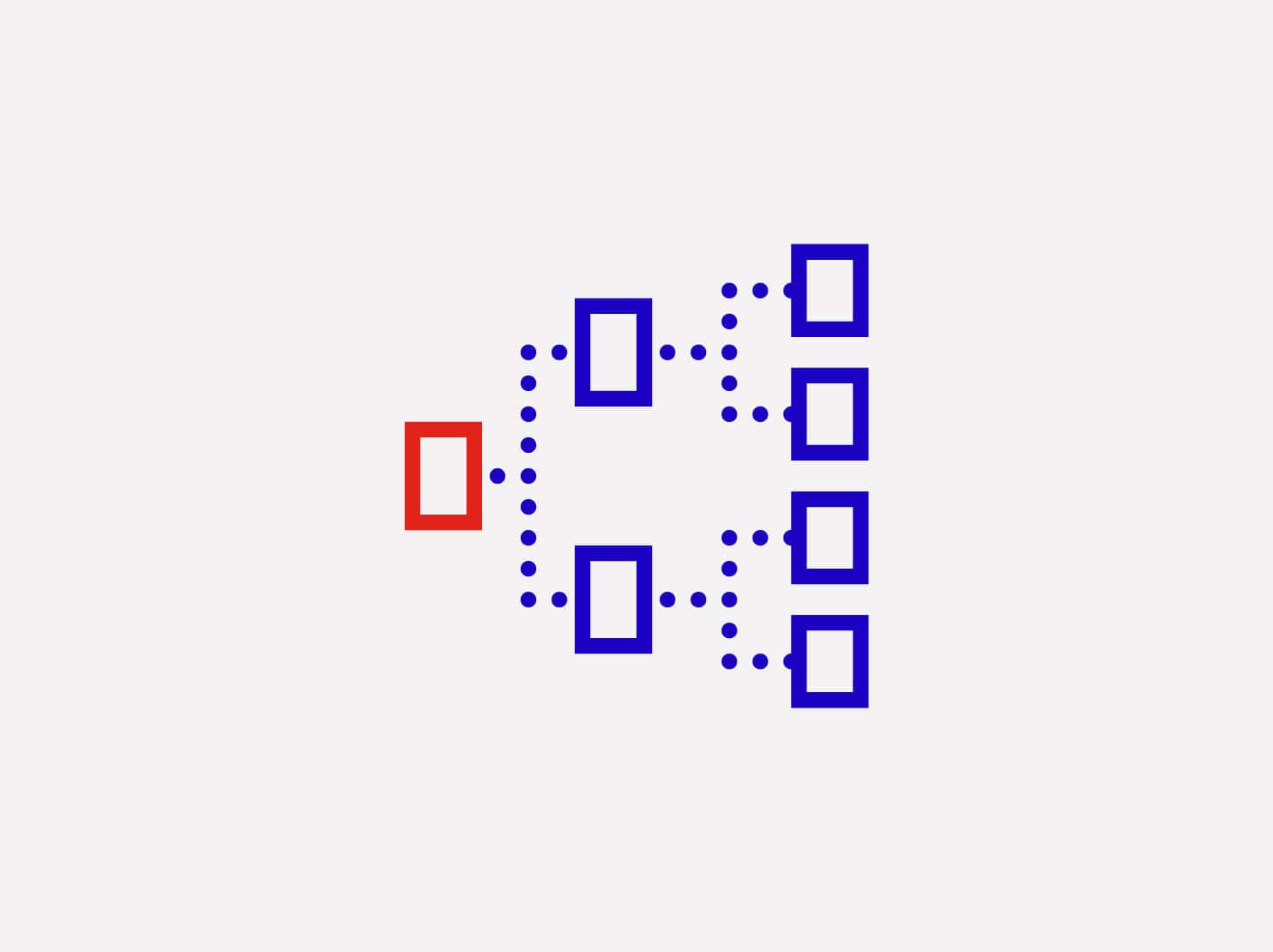
The actual problem
Math is a tool that helps solve problems and analyze possible scenarios. In order to do so, however, the actual problem needs to come into focus. Part of our job and skillset regards this exactly, a practice we call “problem framing”. Imagine being back in high school and brilliantly solving a problem from your textbook, only to later find out that the problem that needed solving was actually on the next page. It’s a waste of energy! So we try to make sure that never happens.
Framing the problem, or — more broadly speaking — aligning business strategy with data (or AI) strategy, is a problem in and of itself, inextricably intertwined with the one mentioned above. There are also applications of math whose goal is precisely to “find the problem”, but in that case the approach is a little different.
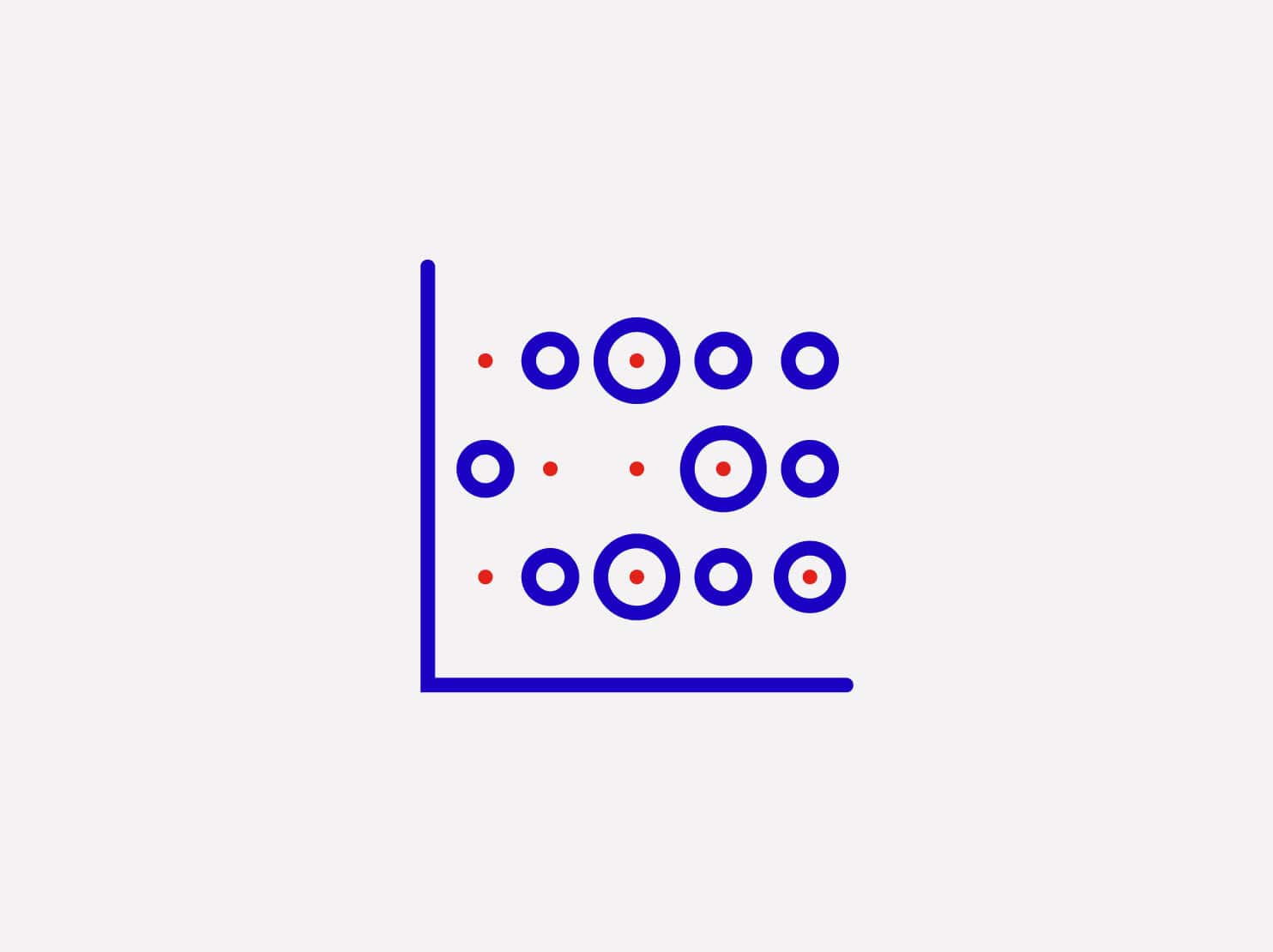
Data
Speaking of data, let’s make something clear. For us, at MAIZE, “data” is any kind of information that can be fed to a machine. Images are data, text is data, sound is data, an Excel sheet is data, a receipt is data. Even the time you are spending reading this piece can be quantified and turned into usable data.
These examples represent both structured and unstructured data; but it’s all data nonetheless. Data is needed to take advantage of math in business, even if scarce. Companies often don’t use the data at their disposal or fail to adopt processes such as data fusion (or data integration), by which internal data is mixed with information coming from third parties and/or other firms. And so, in accordance with the available data and the problem at hand, the apt tech and the related math are chosen.
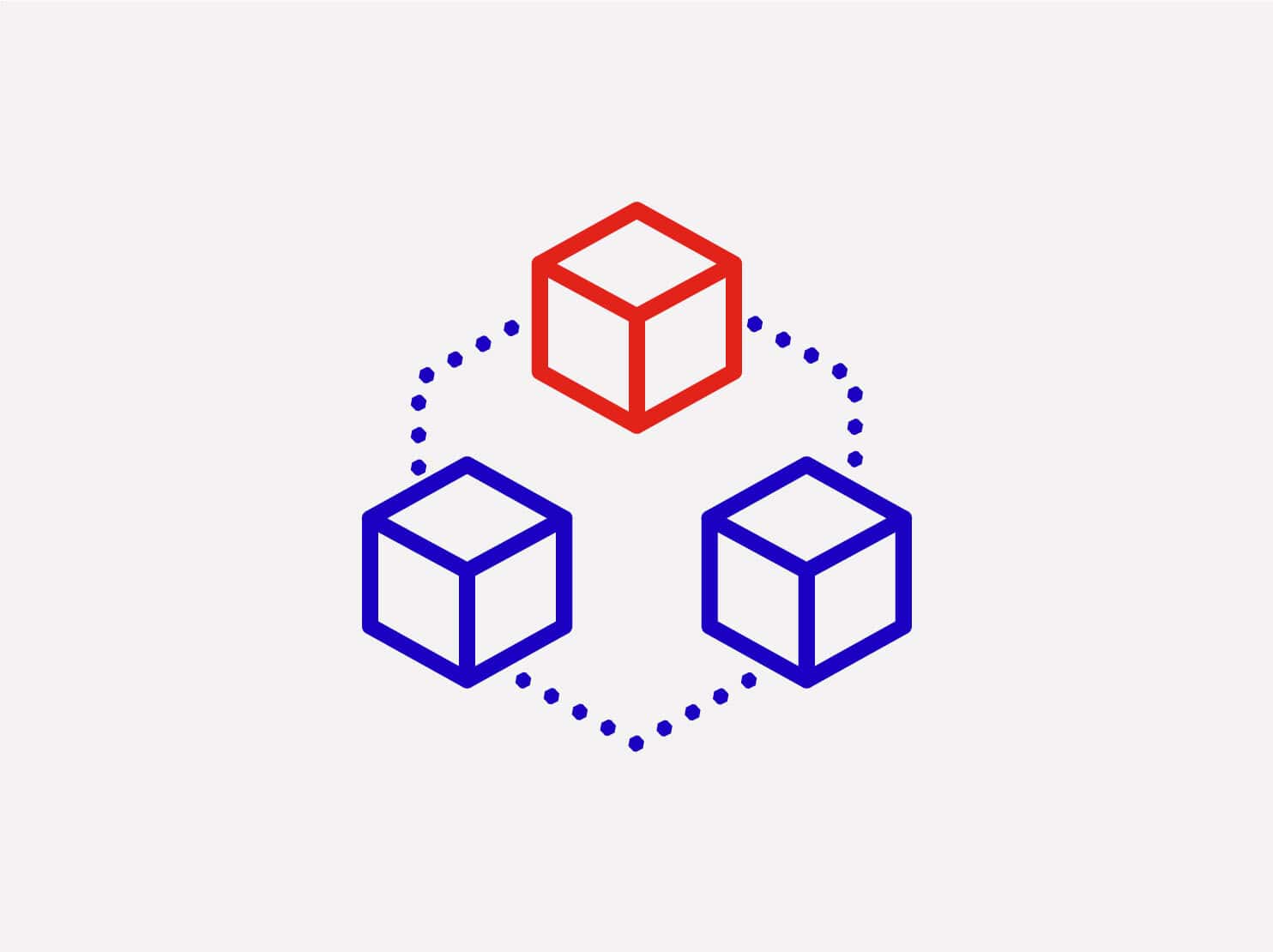
Technology
It used to be the case that people would scribble with pen and paper to solve problems. Now things aren’t that simple anymore. Knowing how to handle the technical aspect of a data project is an essential precondition for a good outcome. Today, actually carrying out this kind of project requires the adoption of cloud architectures, non-relational databases, and many other tools that make tasks and/or their implementation much easier and faster.
Each company has a different approach to technology, and our job is also to understand the most appropriate method for the given problem and the overall technical capabilities of the firm. This thinking allows us to reach optimal, usable solutions and processes that integrate well with the pre-existing organizational order.
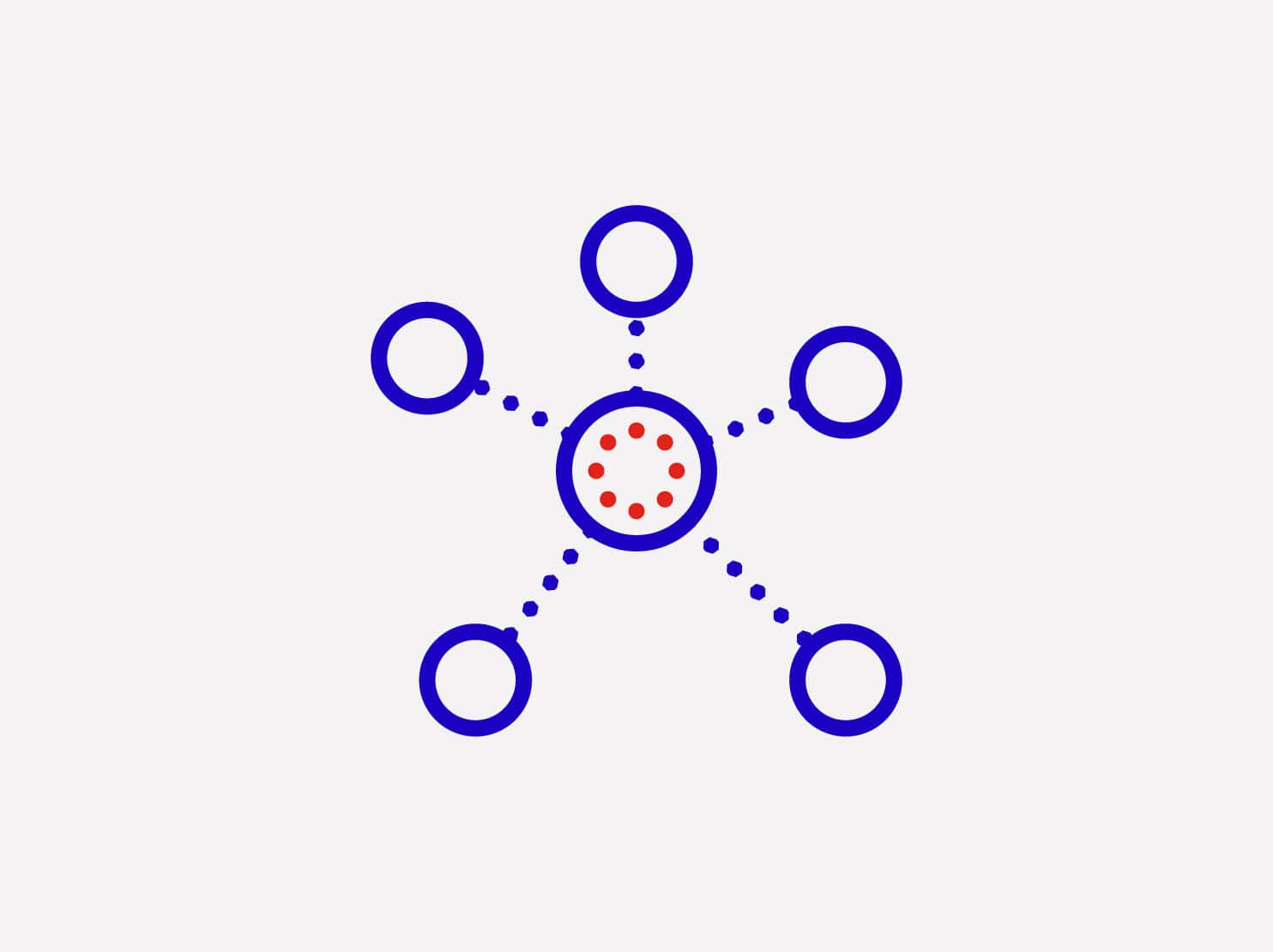
Math itself
Last but not least is, well, the math itself. Theoretical knowledge of models and algorithms is at the core of each project of this kind, and so it is self-evidently crucial. Choosing the wrong model, using the improper dataset to train a neural net, or even just setting some parameters incorrectly can lead to unsatisfactory results. To avoid getting caught in these issues, we at MAIZE have chosen to set up a heterogeneous team of data scientists with skills in both statistics and modeling — a team that’s able to look at each problem from different points of view and come together with an optimal approach.
As is often the case with mathematics, here we have purposely simplified the problem to create a generic model. Each case, however, needs specific analyses and a singular focus on itself. Still, something is universally applicable: even for those of us who believe in this approach and see its positive results on the companies who adopt it every day, the sense of wonder Wigner used to talk about remains.
Seeing an algorithm capable of predicting workplace accidents based on nothing but text reports, just to make one example, is still quite astonishing. And so is the idea that a potentially endless number of scenarios to reflect upon (and all their implications) are but a click away.