What are deep fakes?
With this technology around, you might have to not believe everything you see.
by MAIZE
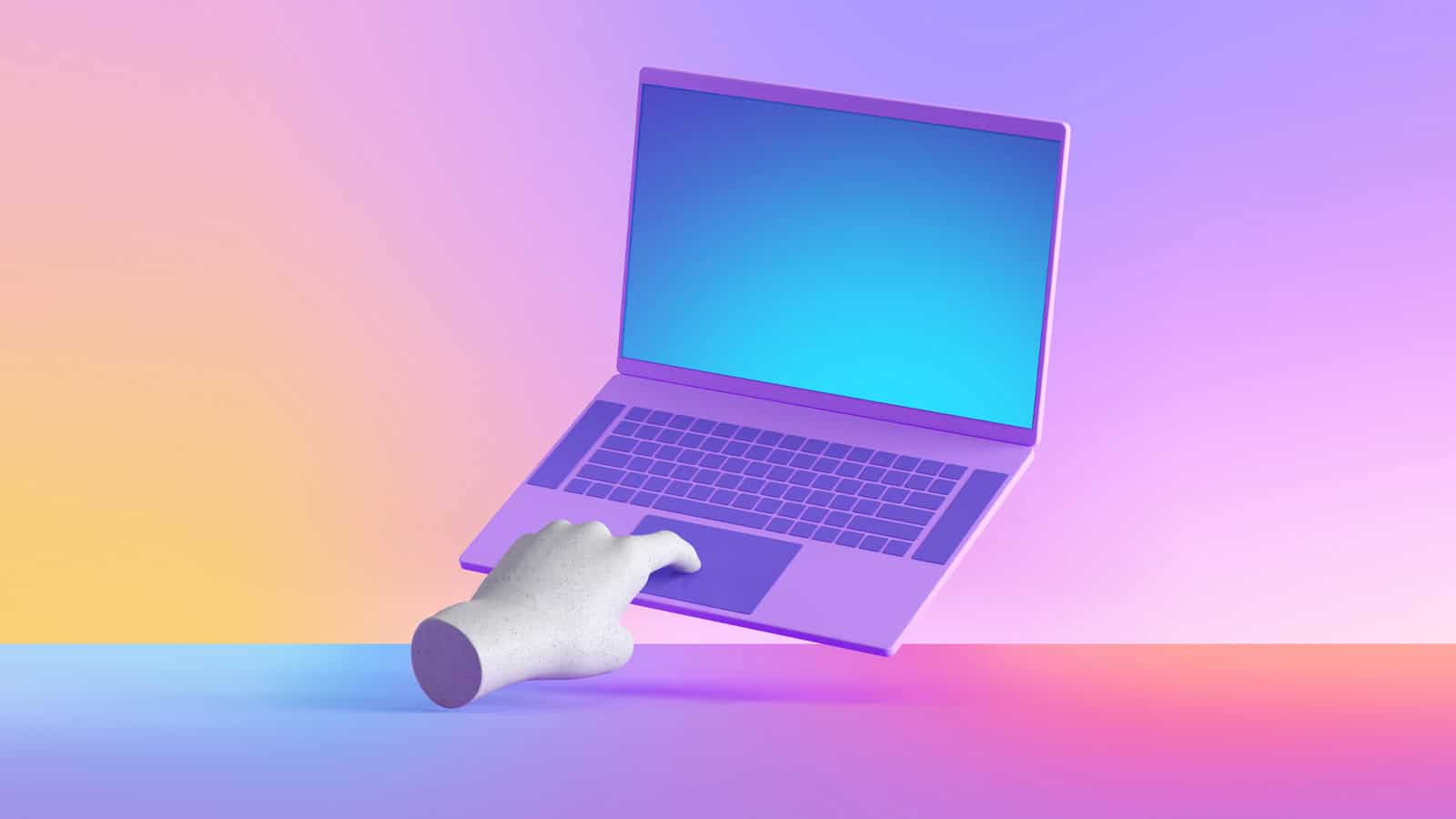
Deep fake, a portmanteau of “deep learning” and “fake”, is an artificial intelligence-based human image synthesis technique. It is used to combine and superimpose existing images and videos onto source images or videos.
For the past year, deep fakes have been picking up traction in the news as people have become more aware of a technology that has been described by researchers at New York University as the “menace on the horizon”. But what has facilitated this fear? Is it warranted? Why did Vice’s Motherboard feel the need to maturely report that: “We are truly fucked”? Let’s find out.
Whilst digital forgery is nothing new, deep fakes use computer programs which harness artificial intelligence in creating what is arguably a watershed moment in how far we have come in opening up the doors of deception.
Deep fakes are created by a machine learning technique called a “generative adversarial network” (GAN). Invented initially by student Ian Goodfellow in 2014 as a way to algorithmically generate new types of data out of existing data sets, this multi-use technology was released onto the internet last by Reddit user ‘deepfakes’ who made the algorithm available using open-source code late last year. Reddit would go on to ban ‘deepfakes’, but it proved too little too late: the technology had already spread, which is where things get a bit more interesting.
You see, deep fakes can recognize patterns in how a person behaves through audio or video recordings of them thanks to the process known as deep learning. This doctored content can then be seamlessly blended with other content as more elements are added. To add to the manipulation, voice-cloning technology – which breaks down audio recordings into bitesized half syllable chunks – can then create a fake dialogue that replicates the person depicted in the video. This voice-cloning technology was also used to create voice assistants such as Apple’s Siri and Amazon’s Alexa.
The implications of this technology are obvious, with the rise of fake news, deep fakes add another layer of lies which can be used to misinform and manipulate individuals into thinking that certain individuals have said or done something which they have not. The most common case in point for the time being is on the seedier side of application: with deep fakes up until now been mostly used for creating pornographic videos of celebrities by mapping their faces onto old pornographic material. However this is just the tip of a very illicit iceberg, and fears that this technology can be used even more nefariously still are proving to be well grounded indeed.
An example of a deep fake video.
As comedian-cum-director, Jordan Peele clearly demonstrated upon the release of his own deep fake, one of former President Obama. Now viewed over 5 million times, it shows the former president referring to his predecessor Donald Trump in an obscene fashion. The deep fake took over 56 hours of sample recordings to create, with the bigger the library of content the deep learning algorithm has to work with, the more realistic the result will be. Apple recorded 10 to 20 hours of speech to create Siri, and, according to a number of reports, voice clones can be made from little more than a few seconds of material. Toby Abel, from AI firm Krzana, described to the BBC the challenges that lie ahead: “The insidious danger of mass amounts of fake news is that we don’t know what to believe”, he said. “I don’t think we have yet got to a point where we know how to handle this”.
Not only is this technology becoming more ubiquitous by the day, with apps such as FakeApp (now removed from the internet) already promoting its popularity, but it is also now the centerpiece of a new technological arms race as academics and researchers try to tame this technology before bad actors do. Researchers at Carnegie Mellon University recently created a system that can transfer characteristics, such as facial expressions, from a video of one person to a synthesized image of another. Whilst China’s Baidu and a handful of startups such as Lyrebird and iSpeech have been selling voice cloning for commercial use in human-machine interfaces.
So how can this problem be contained? For the time being, it seems unlikely. Only six months ago, the potency of this technology in spreading disinformation was made clear when a small left-leaning Belgian political party, Socialistische Partij Anders (sp.a) posted a bombastic deep fake of Donald Trump across their channels, provoking hundreds of Belgians to express anger at their neighbors across the pond. “It is clear from the lip movements that this is not a genuine speech by Trump”, a spokesperson for sp.a told Politico in an exercise in damage control. Apparently not.
All is not lost, however, and researchers have identified certain cues that could indicate a video is a deep fake – with jerky movements and lack of blinking being tell-tale signs. It is true that such details can be missed by viewers, but one would hope that as these videos permeate our lives more and more, the populace will wise-up to what is and is not real (just as there are many more people today than in the past who can identify a photoshopped image for example). It is also true that by increasing the saturation of a video of a person, it is possible to detect the subject’s pulse from the almost invisible change in facial skin. In the case of deep fakes, this is not the case, and the truth is shown.
There is, of course, two sides to every technological coin. And with deep fakes, this is no different. There are of course some positive applications to this technology. One example is the Scottish firm CereProc, who creates digital voices for people who lose their own through disease. Vocal cloning can also serve an educational purpose by recreating the sound of historical figures, just as North Carolina State University did when they synthesized one of Martin Luther King Jr.’s unrecorded speeches.
For now anyway, the cons far outweigh the pros, and, unfortunately, the fact remains that the type of machine learning that produces deep fakes is difficult to reverse and thus detect. For now, all that individuals on the ground can hope for in countering this technology would be to remain vigilant. Moving forward, it would be wise to update that age-old maxim: don’t believe everything you read. Instead, welcome to a world that is home to a new saying: don’t believe everything you see.